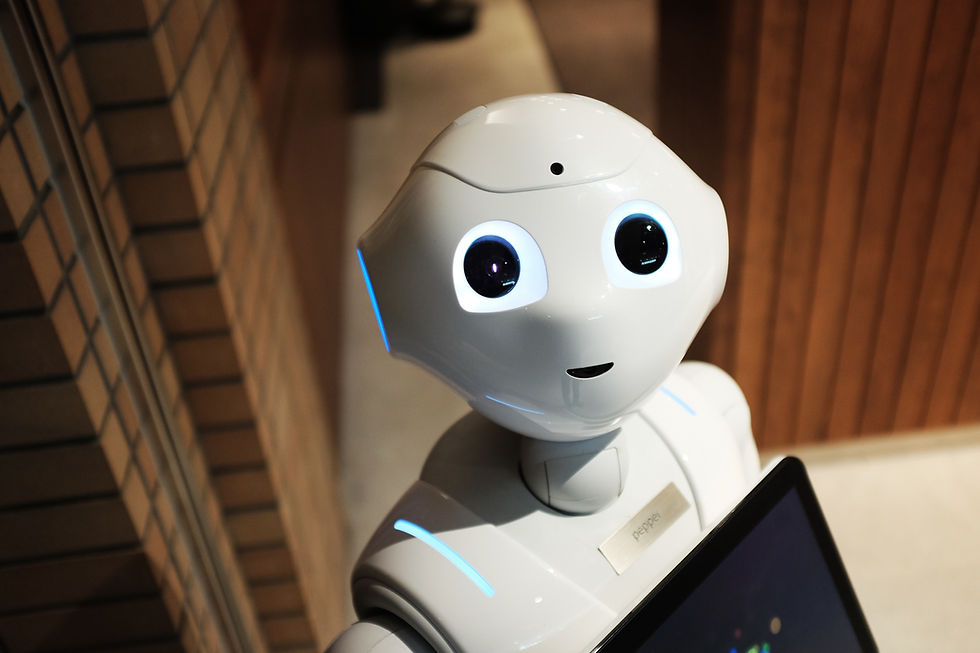
Artificial Intelligence (AI) and Machine Learning (ML) has seen tremendous advances in recent years, catalyzed by growth in datasets, computing power, algorithms, and customized hardware. But what is the future of AI & machine learning in the age of generative AI? Existing traditional AI/ML capabilities remain narrow, leading to cycles of inflated expectations and disillusionment. Breakthroughs in Generative AI like ChatGPT enthral the public imagination but lack flexibility for the real world. How do we chart a measured course to AI's beneficial integration across industries?
Gartner's 2023 Hype Cycle provides grounding, with technologies like generative AI cresting at the "Peak of Inflated Expectations." This phase features unchecked enthusiasm, soaring investment, and exaggerated claims of human-level AI. As limitations surface over 2-5 years, a "Trough of Disillusionment" follows per Gartner's model. Steady real-world deployment continues, guiding ascent up the "Slope of Enlightenment."
On this slope, iterative refinements solve earlier issues and hone value over 5-10 years. We see this today with machine vision in manufacturing or virtual assistants in customer service. Such applications will eventually plateau at widespread productivity. But artificial general intelligence able to replicated multifaceted human cognition likely remains distant.
Key Factors Shaping the Pace and Limits of Progress
- Data: More high-quality, diverse training data powers better performance. However, certain capabilities may always need real-world knowledge and reasoning versus pure data analytics.
- Compute: Larger datasets and models drive exponential demands for processing power and efficiency optimizations like specialized TPU chips. But returns diminish as costs soar into the billions.
- Algorithms and Architecture: Approaches like deep learning, reinforcement learning, neuro-symbolic AI, and modular components can enhance abilities. However new paradigms may be necessary to achieve further major leaps rather than incremental gains.
- Applications: Some use cases like computer vision have crossed the chasm into commercial viability and adoption. Others like AI-synthesized media face unclear mainstream demand despite technical advances.
- Regulation: Governance guardrails can address risks around bias, misuse of AI, and economic impacts like job loss. However, regulations may also stifle innovation if poorly balanced.
- Hardware: Dedicated AI accelerators and speculative technologies like quantum computing could significantly boost capabilities once technical barriers are solved. But feasibility remains distant.
Realistic Near-Term Opportunities by Industry
While true artificial general intelligence remains years if not decades away, narrowly focused AI applications are primed to transform many sectors over the next 5-10 years:
Healthcare
- Clinical decision support systems for diagnosis, treatment recommendations, and patient risk stratification can expand access and quality of care. The global healthcare AI market could reach $45 billion by 2026.
- Robotic surgical assistants can conduct routine tasks, freeing doctors to focus on complex decision making. One study found a 52% reduction in length of stay with AI-assisted surgery.
- Medical imaging analysis like screening radiology scans speeds workflows. AI could take over 30% of a radiologist's workload according to Stanford researchers.
Finance
- Automating loan approval, fraud detection, customer service agents and other analytics with AI could reduce costs by 22% or over $1 trillion per year according to McKinsey.
- Investment and wealth management powered by AI advice platforms is projected to grow to over $20 billion in assets under management globally by 2024.
- Compliance monitoring and surveillance tools enabled by AI can better detect fraud, money laundering, and malicious insider activity.
Manufacturing
- Computer vision systems for quality control, predictive maintenance algorithms, and supply chain analytics can drive the smart factory market to $740 billion by 2027 per MarketsandMarkets.
- Generative design AI can autonomously engineer new product innovations and optimize designs for efficiency and sustainability.
- Collaborative robots enhanced with computer vision and AI planning can work safely alongside humans, improving productivity.
Retail
- Product recommendation engines, demand forecasting, real-time inventory optimization, and customized promotions can be enhanced by AI, with 60% of retailers planning significant investment by 2022.
- Checkout-free technologies like Amazon Go use computer vision and sensor fusion to enable grab-and-go retail, improving convenience.
- Predictive analytics helps retailers tailor marketing, manage risk, and reduce churn by understanding customer behavior.
Transportation
- Autonomous vehicles, trucks, forklifts, and last-mile delivery bots enhanced by computer vision and AI planning aim to make logistic operations safer and more efficient. McKinsey forecasts AI adoption in freight transportation could deliver over $500 billion in annual savings by 2027.
- Route optimization algorithms minimize fuel costs and emissions for transportation companies. Machine learning also enables smoother traffic prediction and control.
- AI image recognition techniques can automate tasks like sign and obstruction detection to reduce risks and improve road safety.
The Future of Work
While AI will displace many jobs, its net impact on employment remains complex. Human roles focused on prediction, data processing, and repetitive tasks face automation. But many occupations will incorporate AI augmentation rather than outright replacement. Business leaders should plan to retrain staff for working alongside AI tools versus struggling against them. Policy makers also need to invest in education reform, job transition programs, and social safety nets to smooth economic displacement.
With prudent governance and implementation respecting human dignity over profit motives alone, AI can enhance knowledge, creativity, and opportunity throughout society. Tracking measured progress along Gartner's maturity cycle offers perspective for enterprises navigating AI's emergence across industries. Leaders should eschew hype while monitoring incremental advancements applicable to real business needs. In time, the transformative potential of artificial intelligence can be realized through strategic adoption and an ethos of augmentation over automation of human labor.
Conclusion
In summary, while hype peaks, AI has a long runway of incremental integration into practical use cases versus AGI speculation. It will permeate more of society and industry, creating opportunities but requiring prudent implementation. Tracking this cycle provides perspective on navigating AI’s emergence.
For businesses, measured strategies are advisable:
- Evaluate augmenting people with narrow AI rather than full automation.
- Audit internal data and pipelines for quality and relevance to use cases.
- Incorporate ethics reviews into deployment plans.
- Discount radical predictions; monitor incremental progress.
The future of AI promises to be a prolonged journey of incremental integration into society and industry, not an overnight revolution. With patient cultivation instead of hype, earnest governance, and a focus on enhancing rather than replacing human potential, the benefits can outweigh the risks. AI remains a technology with immense potential to uplift lives if carefully guided up the Slope of Enlightenment.
AI will reshape aspects of life and work over the next decade but likely more as a partner than replacement. With realistic outlooks, smart adoption, and proactive governance, we can maximize its benefits and minimize unwanted consequences. AI remains a technology with immense potential if carefully nurtured up the Slope of Enlightenment.
Comments